As autonomous autos (AVs) edge nearer to widespread adoption, a major problem stays: bridging the communication hole between human passengers and their robotic chauffeurs. Whereas AVs have made outstanding strides in navigating complicated highway environments, they usually wrestle to interpret the nuanced, pure language instructions that come so simply to human drivers.
Enter an revolutionary examine from Purdue College’s Lyles Faculty of Civil and Building Engineering. Led by Assistant Professor Ziran Wang, a crew of engineers has pioneered an revolutionary strategy to reinforce AV-human interplay utilizing synthetic intelligence. Their resolution is to combine massive language fashions (LLMs) like ChatGPT into autonomous driving methods.’
The Energy of Pure Language in AVs
LLMs signify a leap ahead in AI’s capacity to grasp and generate human-like textual content. These subtle AI methods are educated on huge quantities of textual knowledge, permitting them to know context, nuance, and implied that means in ways in which conventional programmed responses can’t.
Within the context of autonomous autos, LLMs supply a transformative functionality. In contrast to typical AV interfaces that depend on particular voice instructions or button inputs, LLMs can interpret a variety of pure language directions. This implies passengers can talk with their autos in a lot the identical method they might with a human driver.
The enhancement in AV communication capabilities is important. Think about telling your automobile, “I am working late,” and having it robotically calculate probably the most environment friendly route, adjusting its driving type to securely decrease journey time. Or take into account the flexibility to say, “I am feeling a bit carsick,” prompting the automobile to regulate its movement profile for a smoother trip. These nuanced interactions, which human drivers intuitively perceive, change into attainable for AVs by way of the mixing of LLMs.
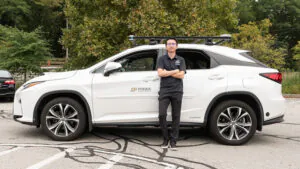
Purdue College assistant professor Ziran Wang stands subsequent to a take a look at autonomous automobile that he and his college students geared up to interpret instructions from passengers utilizing ChatGPT or different massive language fashions. (Purdue College photograph/John Underwood)
The Purdue Examine: Methodology and Findings
To check the potential of LLMs in autonomous autos, the Purdue crew performed a sequence of experiments utilizing a degree 4 autonomous automobile – only one step away from full autonomy as outlined by SAE Worldwide.
The researchers started by coaching ChatGPT to answer a spread of instructions, from direct directions like “Please drive quicker” to extra oblique requests similar to “I really feel a bit movement sick proper now.” They then built-in this educated mannequin with the automobile’s present methods, permitting it to think about components like site visitors guidelines, highway situations, climate, and sensor knowledge when deciphering instructions.
The experimental setup was rigorous. Most checks had been performed at a proving floor in Columbus, Indiana – a former airport runway that allowed for secure high-speed testing. Further parking checks had been carried out within the lot of Purdue’s Ross-Ade Stadium. All through the experiments, the LLM-assisted AV responded to each pre-learned and novel instructions from passengers.
The outcomes had been promising. Contributors reported considerably decrease charges of discomfort in comparison with typical experiences in degree 4 AVs with out LLM help. The automobile persistently outperformed baseline security and luxury metrics, even when responding to instructions it hadn’t been explicitly educated on.
Maybe most impressively, the system demonstrated a capability to be taught and adapt to particular person passenger preferences over the course of a trip, showcasing the potential for actually customized autonomous transportation.
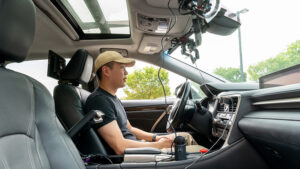
Purdue PhD pupil Can Cui sits for a trip within the take a look at autonomous automobile. A microphone within the console picks up his instructions, which massive language fashions within the cloud interpret. The automobile drives in line with directions generated from the massive language fashions. (Purdue College photograph/John Underwood)
Implications for the Way forward for Transportation
For customers, the advantages are manifold. The power to speak naturally with an AV reduces the educational curve related to new know-how, making autonomous autos extra accessible to a broader vary of individuals, together with those that may be intimidated by complicated interfaces. Furthermore, the personalization capabilities demonstrated within the Purdue examine counsel a future the place AVs can adapt to particular person preferences, offering a tailor-made expertise for every passenger.
This improved interplay may additionally improve security. By higher understanding passenger intent and state – similar to recognizing when somebody is in a rush or feeling unwell – AVs can alter their driving habits accordingly, doubtlessly decreasing accidents attributable to miscommunication or passenger discomfort.
From an business perspective, this know-how may very well be a key differentiator within the aggressive AV market. Producers who can supply a extra intuitive and responsive person expertise could acquire a major edge.
Challenges and Future Instructions
Regardless of the promising outcomes, a number of challenges stay earlier than LLM-integrated AVs change into a actuality on public roads. One key problem is processing time. The present system averages 1.6 seconds to interpret and reply to a command – acceptable for non-critical eventualities however doubtlessly problematic in conditions requiring speedy responses.
One other important concern is the potential for LLMs to “hallucinate” or misread instructions. Whereas the examine included security mechanisms to mitigate this threat, addressing this problem comprehensively is essential for real-world implementation.
Trying forward, Wang’s crew is exploring a number of avenues for additional analysis. They’re evaluating different LLMs, together with Google’s Gemini and Meta’s Llama AI assistants, to check efficiency. Preliminary outcomes counsel ChatGPT at the moment outperforms others in security and effectivity metrics, although revealed findings are forthcoming.
An intriguing future route is the potential for inter-vehicle communication utilizing LLMs. This might allow extra subtle site visitors administration, similar to AVs negotiating right-of-way at intersections.
Moreover, the crew is embarking on a undertaking to review massive imaginative and prescient fashions – AI methods educated on pictures moderately than textual content – to assist AVs navigate excessive winter climate situations widespread within the Midwest. This analysis, supported by the Middle for Linked and Automated Transportation, may additional improve the adaptability and security of autonomous autos.
The Backside Line
Purdue College’s groundbreaking analysis into integrating massive language fashions with autonomous autos marks a pivotal second in transportation know-how. By enabling extra intuitive and responsive human-AV interplay, this innovation addresses a vital problem in AV adoption. Whereas obstacles like processing velocity and potential misinterpretations stay, the examine’s promising outcomes pave the way in which for a future the place speaking with our autos may very well be as pure as conversing with a human driver. As this know-how evolves, it has the potential to revolutionize not simply how we journey, however how we understand and work together with synthetic intelligence in our day by day lives.